Document Type
Article
Publication Date
6-2024
Original Citation
White B,
Woo XY,
Koc S,
Sheridan T,
Neuhauser S,
Wang S,
Evrard YA,
Chen L,
Foroughi Pour A,
Landua JD,
Mashl RJ,
Davis SR,
Fang B,
Rosa MG,
Evans KW,
Bailey MH,
Chen Y,
Xiao M,
Rubinstein J,
Sanderson B,
Lloyd M,
Domanskyi S,
Dobrolecki LE,
Fujita M,
Fujimoto J,
Xiao G,
Fields RC,
Mudd JL,
Xu X,
Hollingshead MG,
Jiwani S,
Acevedo S,
,
Davis-Dusenbery BN,
Robinson P,
Moscow JA,
Doroshow JH,
Mitsiades N,
Kaochar S,
Pan C,
Caravajal-Carmona LG,
Welm AL,
Welm BE,
Govindan R,
Li S,
Davies MA,
Roth JA,
Meric-Bernstam F,
Xie Y,
Herlyn M,
Ding L,
Lewis MT,
Bult C,
Dean DA,
Chuang J.
A Pan-Cancer Patient-Derived Xenograft Histology Image Repository with Genomic and Pathologic Annotations Enables Deep Learning Analysis Cancer Research. 2024;84(13):2060-72.
Keywords
JGM, JMG
JAX Source
Cancer Research. 2024;84(13):2060-72.
DOI
https://doi.org/10.1158/0008-5472.Can-23-1349
Grant
his project has been funded in whole or in part with funds from the National Institutes of Health (NCI U24-CA224067, NCI U54-CA224083, NCI U54- CA224070, NCI U54-CA224065, NCI U54- CA224076, NCI U54-CA233223, NCI U54-CA233306, NCI R01-CA089713); National Cancer Institute; National Insti- tutes of Health (HHSN261201400008C, HHSN261201500003I, under contract HHSN261200800001E); and the Cancer Prevention and Research Initiative of Texas (CPRIT) (RP220646).
Abstract
Patient-derived xenografts (PDX) model human intra- and intertumoral heterogeneity in the context of the intact tissue of immunocompromised mice. Histologic imaging via hematoxylin and eosin (H&E) staining is routinely performed on PDX samples, which could be harnessed for computational analysis. Prior studies of large clinical H&E image repositories have shown that deep learning analysis can identify intercellular and morphologic signals correlated with disease phenotype and therapeutic response. In this study, we developed an extensive, pan-cancer repository of >1,000 PDX and paired parental tumor H&E images. These images, curated from the PDX Development and Trial Centers Research Network Consortium, had a range of associated genomic and transcriptomic data, clinical metadata, pathologic assessments of cell composition, and, in several cases, detailed pathologic annotations of neoplastic, stromal, and necrotic regions. The amenability of these images to deep learning was highlighted through three applications: (i) development of a classifier for neoplastic, stromal, and necrotic regions; (ii) development of a predictor of xenograft-transplant lymphoproliferative disorder; and (iii) application of a published predictor of microsatellite instability. Together, this PDX Development and Trial Centers Research Network image repository provides a valuable resource for controlled digital pathology analysis, both for the evaluation of technical issues and for the development of computational image–based methods that make clinical predictions based on PDX treatment studies. Significance: A pan-cancer repository of >1,000 patient-derived xenograft hematoxylin and eosin–stained images will facilitate cancer biology investigations through histopathologic analysis and contributes important model system data that expand existing human histology repositories.
Creative Commons License
This work is licensed under a Creative Commons Attribution 4.0 International License.
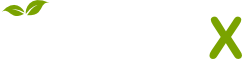
- Citations
- Citation Indexes: 1
- Usage
- Downloads: 28
- Abstract Views: 10
- Captures
- Readers: 6
- Mentions
- News Mentions: 1