Document Type
Article
Publication Date
6-1-2024
Original Citation
Mitchell J,
Chi Y,
Thapa M,
Pang Z,
Xia J,
Li S.
Common data models to streamline metabolomics processing and annotation, and implementation in a Python pipeline. PLoS Comput Biol. 2024;20(6):e1011912.
Keywords
JGM, Metabolomics, Software, Computational Biology, Lipidomics, Chromatography, Liquid, Tandem Mass Spectrometry, Programming Languages, Humans
JAX Source
PLoS Comput Biol. 2024;20(6):e1011912.
ISSN
1553-7358
PMID
38843301
DOI
https://doi.org/10.1371/journal.pcbi.1011912
Grant
This work was supported by the National Institutes of Health (U01CA235493, R01AI149746, R01AI149746S1, UM1HG012651 to SL). The funders had no role in study design, data collection and analysis, decision to publish, or preparation of the manuscript.
Abstract
To standardize metabolomics data analysis and facilitate future computational developments, it is essential to have a set of well-defined templates for common data structures. Here we describe a collection of data structures involved in metabolomics data processing and illustrate how they are utilized in a full-featured Python-centric pipeline. We demonstrate the performance of the pipeline, and the details in annotation and quality control using large-scale LC-MS metabolomics and lipidomics data and LC-MS/MS data. Multiple previously published datasets are also reanalyzed to showcase its utility in biological data analysis. This pipeline allows users to streamline data processing, quality control, annotation, and standardization in an efficient and transparent manner. This work fills a major gap in the Python ecosystem for computational metabolomics.
Creative Commons License
This work is licensed under a Creative Commons Attribution 4.0 International License.
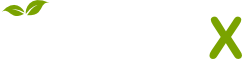
- Citations
- Citation Indexes: 2
- Usage
- Downloads: 5
- Abstract Views: 1
- Captures
- Readers: 18
- Mentions
- News Mentions: 1