Document Type
Article
Publication Date
10-1-2024
Original Citation
Gosai S,
Castro R,
Fuentes N,
Butts J,
Mouri K,
Alasoadura M,
Kales S,
Nguyen T,
Noche R,
Rao A,
Joy M,
Sabeti P,
Reilly S,
Tewhey R.
Machine-guided design of cell-type-targeting cis-regulatory elements. Nature. 2024;634(8036):1211-20.
Keywords
JGM, Animals, Female, Humans, Male, Mice, Cell Line, Deep Learning, Gene Expression Regulation, Genes, Reporter, Genetic Engineering, Genome, Human, Neural Networks, Computer, Organ Specificity, Regulatory Sequences, Nucleic Acid, Cells, Reproducibility of Results, Machine Learning, Computer Simulation, Zebrafish
JAX Source
Nature. 2024;634(8036):1211-20.
ISSN
1476-4687
PMID
39443793
DOI
https://doi.org/10.1038/s41586-024-08070-z
Abstract
Cis-regulatory elements (CREs) control gene expression, orchestrating tissue identity, developmental timing and stimulus responses, which collectively define the thousands of unique cell types in the body1–3 . While there is great potential for strategically incorporating CREs in therapeutic or biotechnology applications that require tissue specificity, there is no guarantee that an optimal CRE for these intended purposes has arisen naturally. Here we present a platform to engineer and validate synthetic CREs capable of driving gene expression with programmed cell-type specificity. We take advantage of innovations in deep neural network modelling of CRE activity across three cell types, efficient in silico optimization and massively parallel reporter assays to design and empirically test thousands of CREs4–8 . Through large-scale in vitro validation, we show that synthetic sequences are more effective at driving cell-type- specific expression in three cell lines compared with natural sequences from the human genome and achieve specificity in analogous tissues when tested in vivo. Synthetic sequences exhibit distinct motif vocabulary associated with activity in the on-target cell type and a simultaneous reduction in the activity of off-target cells. Together, we provide a generalizable framework to prospectively engineer CREs from massively parallel reporter assay models and demonstrate the required literacy to write fit-for-purpose regulatory code.
Creative Commons License
This work is licensed under a Creative Commons Attribution 4.0 International License.
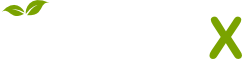
- Citations
- Citation Indexes: 13
- Usage
- Downloads: 10
- Abstract Views: 2
- Captures
- Readers: 95
- Mentions
- Blog Mentions: 1
- News Mentions: 11